Quickly Load CSVs into PostgreSQL Using Python and Pandas
Use Pandas to quickly create and populate a Postgres database
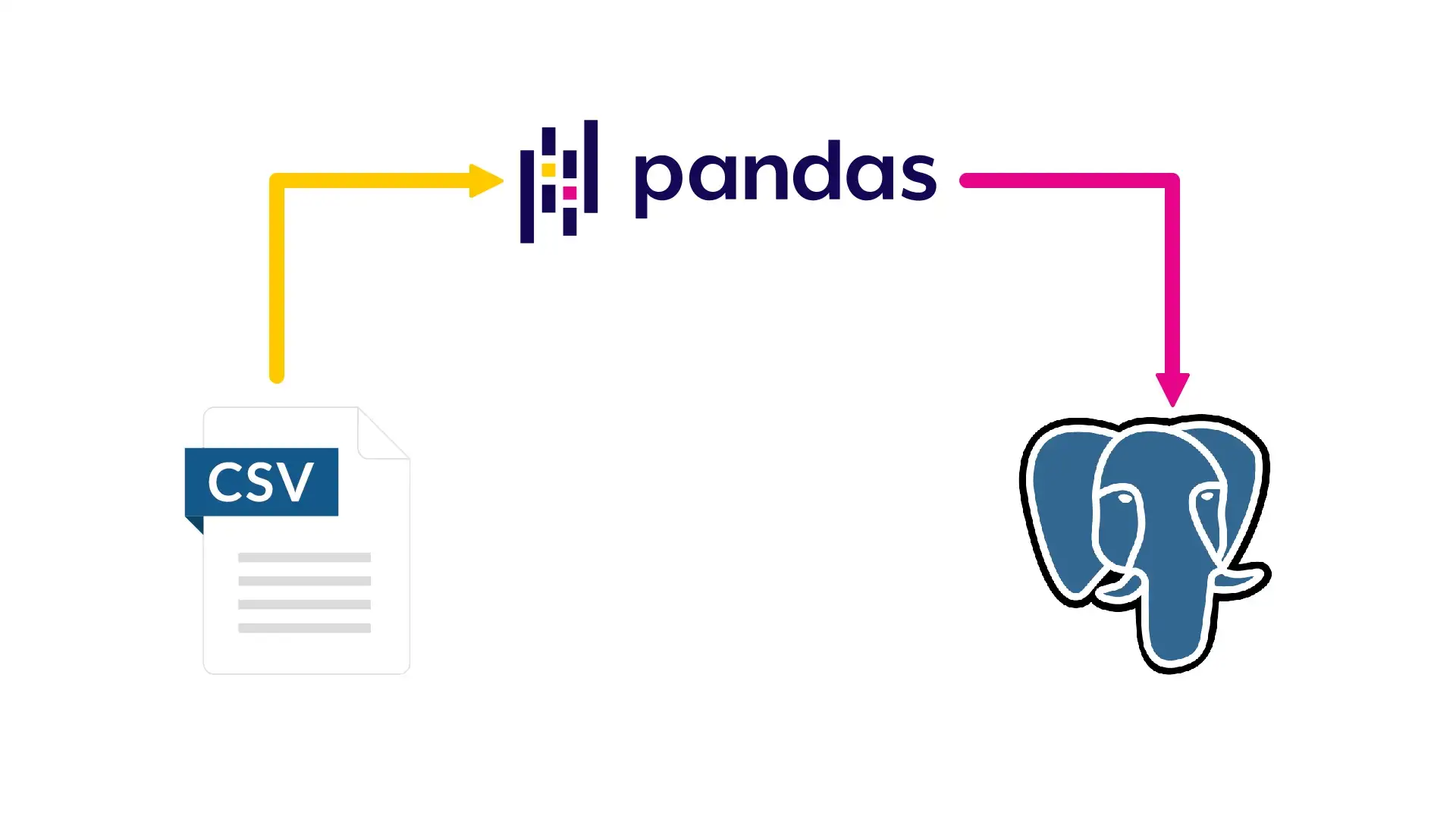
Sometimes it can be kind of a pain to deal with copying CSV data into a Postgres database — especially if you don't want to write out a long schema. Why not let Pandas do all that legwork for you? I'll walk you through a quick example using the Iris dataset (here's a link to the data).
Loading CSV Data Into Postgres with Pandas
Assuming you have a Postgres server up and running, you'll need to either create a database to store your data or use an existing one. Using your preferred Postgres interface (i.e. psql or pgAdmin), you can run the following to create your database…
CREATE DATABASE iris;
Now that you have a database to store your table, you can move over to Python to import your data. You'll need the SQLAlchemy
Python toolkit (if you don't already have it, it can be installed with pip install SQLAlchemy
).
You're going to load your CSV data with Pandas and then use SQLAlchemy to pass the data to Postgres. The SQLAlchemy.create_engine
will need the following 6 pieces of information:
- The DBMS — In this case, that will be "postgres"
- A username for the database
- That user's password
- The hostname for the Postgres server
- The port for the Postgres server (
5432
by default) - The name of the database to store the new table
And it will be formatted as follows:
create_engine("<dbms>://<username>:<password>@<hostname>:<port>/<db_name>")
Here's how that will look in code...
# Imports import pandas as pd from sqlalchemy import create_engine # This CSV doesn't have a header so pass # column names as an argument columns = [ "sepal_length", "sepal_width", "petal_length", "petal_width", "class" ] # Load in the data df = pd.read_csv( "iris.csv", names=columns ) # Instantiate sqlachemy.create_engine object engine = create_engine( 'postgresql://postgres:my_password@localhost:5432/iris' ) # Save the data from dataframe to # postgres table "iris_dataset" df.to_sql( 'iris_dataset', engine, index=False # Not copying over the index )
If your CSV data is too large to fit into memory, you might be able to use one of these two options…
Working with Large Datasets: Option 1
One option would be to use the Pandas chunksize
argument for pd.read_csv
which will return a generator that will iterate through rows of the CSV and yield DataFrames
with the number of rows corresponding to the specified chunksize. Here's what that might look like…
# Imports import pandas as pd from sqlalchemy import create_engine # This CSV doesn't have a header so pass # column names as an argument columns = [ "sepal_length", "sepal_width", "petal_length", "petal_width", "class" ] # Instantiate sqlachemy.create_engine object engine = create_engine( 'postgresql://postgres:my_password@localhost:5432/iris' ) # Create an iterable that will read "chunksize=1000" rows # at a time from the CSV file for df in pd.read_csv("iris.csv",names=columns,chunksize=1000): df.to_sql( 'iris_dataset', engine, index=False, # if the table already exists, append this data if_exists='append', )
Working with Large Datasets: Option 2
Another option (which is a bit more of a hack) would be to load a few rows of data into pandas — enough for it to infer the column datatypes — and then read in the CSV using psql. Here's what that might look like…
# Imports import pandas as pd from sqlalchemy import create_engine # This CSV doesn't have a header so pass # column names as an argument columns = [ "sepal_length", "sepal_width", "petal_length", "petal_width", "class" ] # Load in the data df = pd.read_csv( "iris.csv", names=columns, nrows=1000 # load in the first 1000 rows ) # Instantiate sqlachemy.create_engine object engine = create_engine( 'postgresql://postgres:my_password@localhost:5432/iris' ) # Save the data from dataframe to # postgres table "iris_dataset" df.to_sql( 'iris_dataset', engine, index=False )
Then, once Pandas has created the table you can run the following two lines in psql
in order to delete those few rows added by Pandas and let Postgres re-import the full data...
DELETE FROM iris_dataset; \COPY iris_dataset FROM 'downloads/iris.csv' DELIMITER ',' CSV;
Hopefully that helps and let me know if you have any other suggested techniques!